One paper receives Best Paper Award in WSDM'25
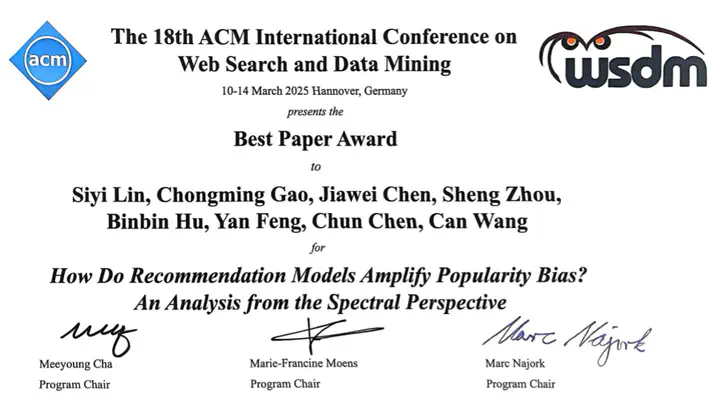
Congratulations to Siyi Lin, Jiawei Chen and all the collaborators for winning the Best Paper Award at the WSDM'25 for their paper “How Do Recommendation Models Amplify Popularity Bias? An Analysis from the Spectral Perspective”.
Congratulations to Siyi Lin, Jiawei Chen and all the collaborators for winning the Best Paper Award at the WSDM'25 for their paper “How Do Recommendation Models Amplify Popularity Bias? An Analysis from the Spectral Perspective”.
The popularity bias in recommendation systems refers to the phenomenon where models optimized on long-tail data inherit and amplify this long-tail effect, leading to recommendations that are increasingly skewed towards popular items. This results in issues such as information silos and the Matthew effect. This work provides a theoretical explanation for the causes of popularity bias in recommendation systems, offering a new perspective for bias analysis and long-tail optimization through a frequency domain approach. Based on this theoretical framework, a simple yet effective new debiasing method is proposed, which significantly outperforms existing methods.
Specifically, this paper elucidates the intrinsic mechanisms by which recommendation models memorize and amplify popularity bias through both theoretical and empirical analyses: 1) Popularity information of items is retained in the primary spectral components of the model’s prediction matrix; 2) Due to the inherent dimensional decay problem in recommendation models, the influence of the primary spectral components is magnified, causing popularity to play a more significant role in predictions.
Building on this analysis, the paper introduces a targeted debiasing method based on spectral norm constraints, demonstrating optimal performance across seven real-world datasets and three testing scenarios.
Paper: https://arxiv.org/pdf/2404.12008 Chinese Introduction: https://mp.weixin.qq.com/s/D9xS_1c3ClMtqO2x62O5yQ